Introductory tutorials
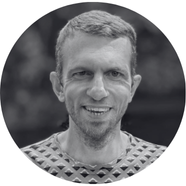
Thomas Akam: Brain architecture for adaptive behaviour
I will give a high-level overview of brain circuits for adaptive behaviour, aimed at an audience with a machine learning background interested in how learning algorithms map onto the functional and anatomical architecture of the brain. I will start with the dopamine system and its primary target the striatum; the poster child for brain reinforcement learning (RL) due to striking resemblance between dopamine neuron activity in many situations and the reward prediction error (RPE) in temporal difference RL. I will discuss how dopamine-mediated synaptic plasticity in the striatum may provide a substrate for temporal difference RL, and then consider the primary inputs and outputs of this system to situate it in the broader brain circuitry of adaptive behaviour. On the output side, the basal ganglia can directly control motor actions via projection to brainstem, but can also gate activity in other brain regions, potentially implementing selection over internal ‘cognitive’ actions. On the input side I will focus on cortex, a hierarchically organised recurrent network, often conceptualised as using unsupervised or self-supervised learning to infer the latent causes of sensory input, and hence provide an suitable state representation for action selection. The top of the cortical hierarchy, frontal cortex, is itself strongly implicated in reward-guided decision making, with neuronal activity that often tracks values or RPEs in decision tasks, raising questions about the respective contributions of unsupervised and reinforcement learning, and of changes in recurrent activity vs synaptic weights, in behavioural adaptation. Finally, I will touch on the hippocampus, a brain structure famous for its roles in episodic memory and the representation of space, which spontaneously generates sequential activity patters that resemble simulated trajectories through the environment, a possible substrate for model-based RL. I will also highlight some tensions between methods used to achieve stable learning in engineered deep RL systems, and those that appear plausible in biological brains, and suggest that aspects of cortico-basal ganglia circuit architecture may be evolution’s solution to achieving stable learning and control under biological constraints.
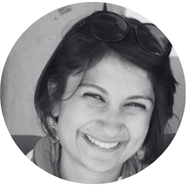
Anna Harutyunyan: Reinforcement learning: an anti-tutorial
In this “anti-tutorial”, we’ll examine the reinforcement learning framework through a critical lens. What are its core assumptions and narratives? What kind of intelligence does it truly model? What questions go unasked, and what answers remain out of reach?
We will then motivate and explore a complementary perspective, grounded in a different ontological starting point, and consider the new lenses it affords.
Finally, we’ll reflect on meta-principles for doing research that deliberately steps outside of its inherited frames.
Advanced tutorials
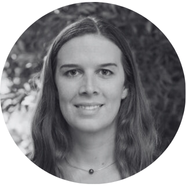
Caroline Charpentier: Leveraging individual differences in RLDM
Individuals differ from one another in how they learn and make decisions, especially in our complex social world. Such heterogeneity isn’t all noise; instead, studying it can offer novel insights into mechanistic subtypes and computational cognitive profiles. In this talk, I will discuss how the field of computational psychiatry has provided a range of methods to characterize heterogeneity, including linking computational model parameters (e.g. learning biases) to transdiagnostic symptom dimensions, and unsupervised clustering to identify subgroups with distinct learning and decision-making strategies that also exhibit distinct symptom profiles. I will also explore recent insights and future directions related to social learning, and the benefits of integrating computational modelling frameworks into the social contexts in which learning and decisions naturally take place. While the talk will primarily focus on how leveraging individual differences can provide critical insights into human cognition and mental health, this approach carries cross-disciplinary relevance for AI systems, which exhibit their own heterogeneity across AI models and must adapt to individual variability in their human users.
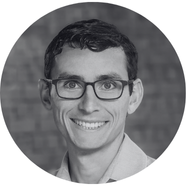
Ben Eysenbach: Self-Supervised Representations and Reinforcement
What fundamentally makes the reinforcement learning (RL) problem difficult is that the space of behaviors is large and complex. This tutorial introduces self-supervised reinforcement learning — a family of methods in which agents autonomously generate their own rewards (e.g., via intrinsic motivation) to learn skills that cover this large space of behaviors. These skills are not programmed in advance, but rather are discovered through exploring and experimenting, without using human demonstrations. By efficiently representing the space of possible behaviors, this set of skills (sometimes called a behavioral foundation model) can be leveraged to rapidly solve new tasks.
Self-supervised RL has become an active area of research over the last decade. This tutorial will start by introducing algorithmic techniques for learning these skills, including ones based on goals and intrinsic rewards. We will then discuss the mathematical underpinnings of skills, and how skills can be used to solve downstream tasks. We will end by highlighting several open problems.
This tutorial assumes a basic familiarity with reinforcement learning, machine learning and probability.